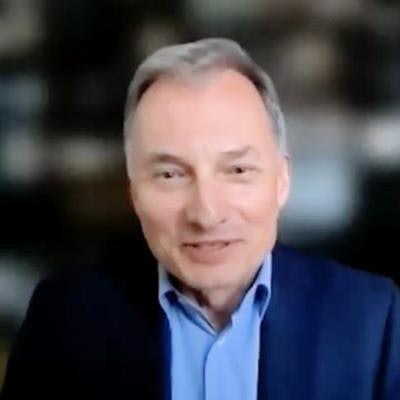
How close are we to implementing artificial intelligence (AI) for lung cancer screening? How can AI assist radiology workflow? Which applications are most exciting? These and other questions were addressed in a webinar about thoracic AI trends on 6 April by an expert panel that included Prof. Mathias Prokop, PhD, head of radiology at Radboud University Medical Center in Nijmegen, the Netherlands, and president of the Dutch Society of Radiology (NVvR).
In this Q&A article, Prokop speaks about the development of AI in thoracic imaging and the areas of special interest to him, such as AI-integrated workstations for automation of tasks, interactions between vendors and radiologists, the future of lung screening, and how to avoid burnout.
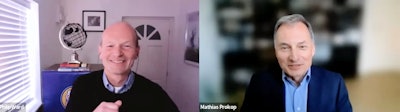
Q: How integral is AI to your own practice?
A: Not as much as I would like it to be. We use AI daily when it comes to image acquisition and reconstruction in CT and MRI. AI-based image reconstruction in CT is done on the vast majority of our scanners, and in MRI, acquisition is driven by AI techniques.
When it comes to the more spectacular and visible AI approaches for the interpretation of images, we only use it all the time in two areas. One of them is bone age investigation from a hand x-ray, which has been used for over seven years and is very simple and works perfectly. We use it in children to determine age and to check their predicted growth. Also, we've started using AI more recently for the automatic classification of breast density, an application that works really well. We have AI installed for mammography interpretation, to determine relevant lesions.
We have also experimented with quite a few AI applications, one for stroke, which we kept for a year and then stopped using as it just didn't do what we wanted it to. Another application is for triaging chest x-rays, so we don't need to read some x-rays for lung nodules anymore. However, this is still in the experimental phase and hasn't changed our practice massively.
Q: As a cardiothoracic imaging specialist, which AI tools excite you the most?
A: I think we're getting closer to seeing fully automatic reading of lung screening CTs. Our research group has been doing amazing work in this area. It will be a few years until it is feasible, and the regulatory process will take a while. We are also seeing some AI integrated into workstations. A lot of the automatic contouring that is done for example for cardiac MRI is now much better than it used to be thanks to deep learning.
In the future, I would like to see more AI for workflow support. That's where we can pay for it and where we need it most. Since our healthcare system needs to remain affordable, AI has to finance itself by allowing us to read scans more quickly, and with less exhaustion and burnout.
Q: How can AI assist radiology workflow?
Bone-age AI is a classic example and has totally changed our workflow. But this is a tiny application. To really make a difference in terms of workflow and costs, you need to focus on something done quite frequently, that costs quite a bit of time, and where you think you can reduce the time and become better and more precise.
In oncology follow-up, I would like to have a workstation that rapidly guides me through the workflow of measuring lesions and helps me find new ones. In addition, it should cover the quick handling of incidental findings, which are usually quite frequent in these patients. As a result, I’d like to end up with a very standardized report without having to leave my PACS workstation environment and without having to dictate anything. These workstations don't exist yet, so there is an opportunity here for clever developers who really interact well with radiologists to look at what we need and help us get it, allowing us to achieve better diagnoses faster. Ultimately, that's the holy grail for me.
Q: How is AI improving cardiac interpretation?
A: AI really has helped us by automatically doing all the segmentations that are necessary with less interaction. We see that the time it takes to do a full evaluation of the heart on MR has really sped up by using AI tools.
AI for cardiac CT has been around for a long time and techniques have improved. Coronary centerline tracking has become better, although the interaction around it is sometimes a bit old-fashioned, so there is some need for updating.
I expect that over the next 10 years, the routine work we have to do as cardiac radiologists will be massively standardized and simplified. AI will automatically populate our reports so that reading cardiac CTs and MR images will become more efficient and faster. How quickly we get there depends on the amount of data that is available to train AI systems. The problem now is that we have systems that are trained on relatively small datasets. The more we are able to collect and share datasets to train AI, the faster it will happen. I think we need five to 10 years to get to that point in cardiac imaging.
Q: Do you have any other advice for AI developers?
A: Focus on workflow. We need good AI performance to ensure better workflow that does not require us to manually correct the system too often, so this is also relevant. This means training your system with relevant cases and nailing down your "use case." One of the reasons we threw out the brain AI for stroke was that it was mainly finding proximal stenoses that radiologists could easily see but was not trained to find M2 stenoses that are more difficult to detect.
Q: One area of uncertainty is what to do when the radiologist disagrees with the output from AI. In such a situation, can AI results be updated?
A: It depends on implementation. For example, in an AI system that detects lung nodules and sends nodule annotations to PACS, you usually cannot change these annotations. It works well if it has a high sensitivity and then helps not to miss lesions. However, if a radiologist disagrees and does not think a marked lesion is a nodule, this has to be clearly stated in the report because even the false positive marks will remain in the PACS, visible for all.
If you want a situation where you can correct output, it means you have to find a way to interact with data. If the data sent to PACS is like an overlay compatible with the overlay functions on your PACS, you could just delete the measurements, but then the AI system would not learn. For the system to learn, you need two-way communication or a workstation in which the AI solution is integrated -- then the user can interact and for example, adjust the segmentation or reject a nodule or add a new nodule. There needs to be integration, either in the PACS for pulling up this type of software or through a standalone workstation that supports this type of workflow.
Q: How do you think AI should be monitored and degradation addressed?
A: Degradation is especially problematic if you have self-learning systems. Distribution shift is also an issue; if there is a change to your patient population, scanner, sequence, or reconstruction, then you can run into trouble. Building something into the software that monitors performance could be helpful, but it doesn't work for all tasks. It would be good to have some more research on that so AI can tell you when it becomes insecure or when the finding itself is outside the range of the training set, for example if the finding is very rare or unusual. In such a situation, the AI output will be more error-prone. These internal error checks can -- and should -- be done more and more, especially when it comes to contouring of cardiac data and also for decision support.
Q: How have you used AI in COVID-19 cases? How is your database advancing?
A: In the first wave, we used CT a lot, and nearly all COVID-19 patients coming into the hospital would get a CT due to a lack of polymerase chain reaction testing. During this phase, we developed an AI system and trained it on these data, and it worked well for scoring cases, for predicting whether or not the patient had COVID, and for quantifying lung involvement.
However, in the subsequent waves, CT became less important, and we don't see that many patients anymore. So we are in a situation where we have a really nice AI system that is not very useful because in practice we only look at sporadic cases.
With regard to the database, we have roughly 1200 cases in it, including follow-up CTs.
Q: Over two years have passed since you reported the results from Dutch-Belgian Randomized Lung Cancer Screening (NELSON) trial. What's the status of the project now?
A: The project is closed, but we're looking more closely at some of the data. For example, can we predict more quickly whether a nodule is malignant or not? Also, why do a lot of the patients still die from lung cancer, despite us finding it? These questions are being examined retrospectively.
Q: How do you avoid burnout and overload?
A: I really like what I do -- it's almost become a hobby -- and that helps. If I think I'm getting overloaded, I just don't do everything any more. I massively triage, and I have a lot of people around who help me function. To work well, I need a good team. If I'm in trouble, there are a lot of people I can talk to, and that helps keep me in balance. My wife also helps me calm down!
I have a few hobbies, but the main priority for avoiding overload is to get things really well organized and to know your limits. That's my secret.
Editor's note: You can watch a recording of the AuntMinnieEurope.com and AuntMinnie.com webinar, "AI Trends in 2022: Thoracic Radiology," free on demand via this link. The other speakers were Prof. Marie-Pierre Revel and Imon Banerjee, PhD. The series of AI webinars was produced by Brian Casey and Erik Ridley of AuntMinnie.com, and sponsored by Bayer.